
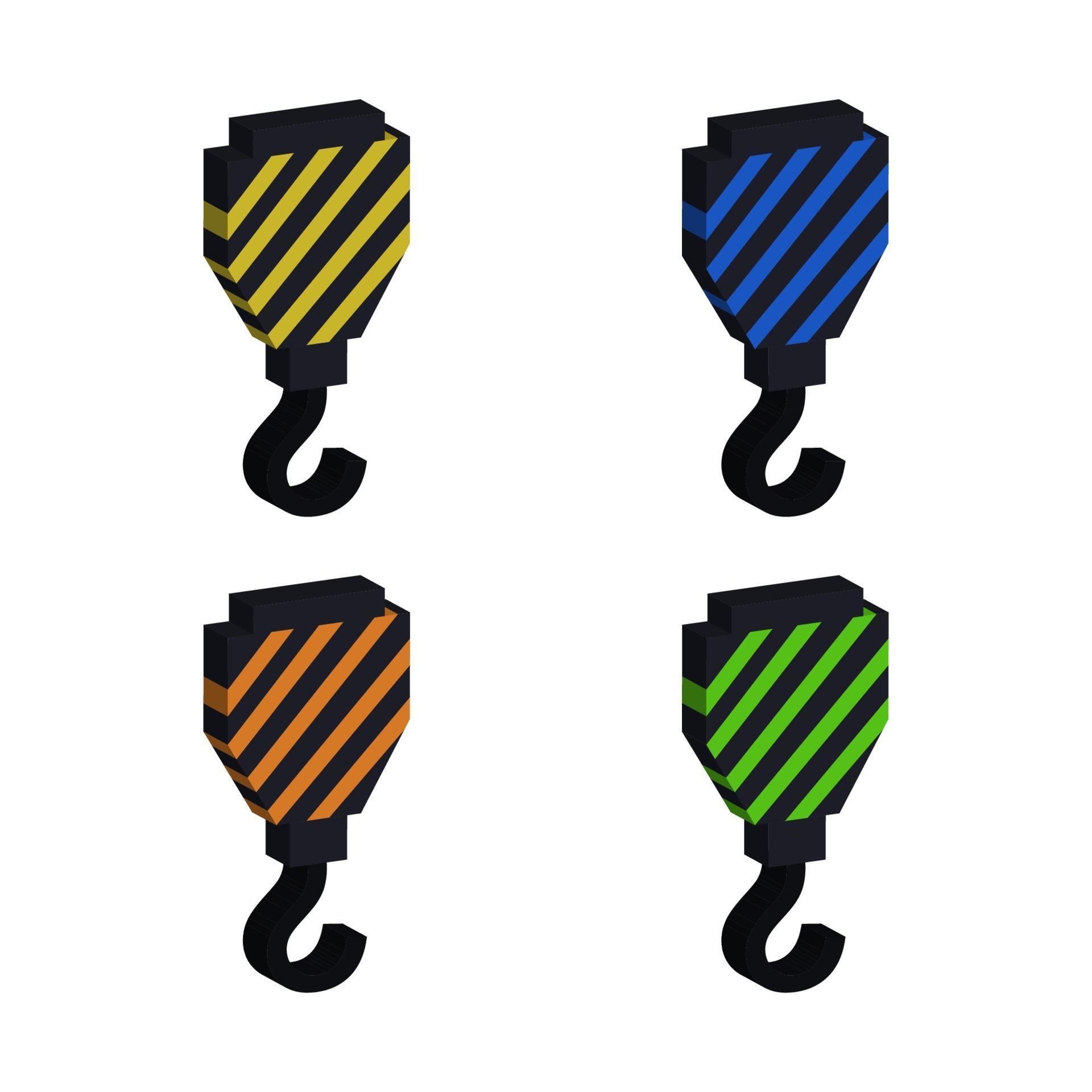
(2016), who replaced the original observed trait data with a smoothed approximation (i.e. These approaches were refined further by Kwak et al. (2014) proposed more computationally efficient regression-based methods. In an attempt to overcome these limitations, while retaining or further increasing statistical power, Moore et al. However, when implemented in its original version, functional mapping is computationally inefficient, particularly when large numbers of TPs and/or markers are analysed ( Wang et al., 2017). Over the last two decades, this methodology has been applied across a range of experimental systems ( Wu and Lin, 2006 Li et al., 2010 Jiang et al., 2015 Xu et al., 2016). Functional mapping based on maximum likelihood ( Ma et al., 2002) was originally proposed to enhance conventional QTL mapping approaches by capturing the additional dynamic information in longitudinal phenotypic data using mathematical functions. The opportunities created by the availability of HTPP data also pose significant analytical challenges in genetic studies aimed at identifying quantitative trait loci (QTLs) in mapping families ( Li and Sillanpaa, 2015 Sun and Wu, 2015) and genomic prediction of complex phenotypic traits in breeding populations ( Cabrera-Bosquet et al., 2012 van Eeuwijk et al., 2019).
#Gancho industrial vector 2d manual#
Because of these limitations and the critical importance of manual or automated data cleaning ( Tardieu et al., 2017), the number of time points (TPs) for which HTPP data are to be extracted and analysed is an important consideration, as is the distribution of these data TPs throughout the growing period of the crop ( Rutkoski et al., 2016 Dreccer et al., 2019). reproductive) are particularly prone to data collection errors ( Dreccer et al., 2019 Rebetzke et al., 2019). Thus, collecting measurements at excessively frequent intervals may not be cost-efficient ( Araus and Kefauver, 2018 Reynolds et al., 2019) and can even result in poorer overall quality of the data set, as certain phenological stages (e.g. alignment, calibration, and segmentation) is demanding, and the collection of data throughout the crop growth cycle of large trials remains onerous ( Araus and Cairns, 2014 Haghighattalab et al., 2016 Pauli et al., 2016). However, even with rapidly increasing computational power and efficiency, image post-processing (i.e. In recent years, field-based high-throughput plant phenotyping (HTPP) tools have been used extensively in crop trials, aiming to reduce or eliminate manual measurements, increase the amount and quality of data for temporally dynamic phenotypes, and, ultimately, translate ‘big data’ collected using various sensors into knowledge ( Rebetzke et al., 2016 Sadeghi-Tehran et al., 2017 Virlet et al., 2017 Araus et al., 2018). These results will inform the development of an integrated, semi-automated analytical pipeline, which will be more broadly applicable to similar data sets in wheat and other crops. Finally, although QTL detection power and predictive ability generally increased with the number of TPs analysed, gains beyond five or 10 TPs chosen based on phenological information had little practical significance. Using spline-smoothed phenotypic data resulted in improved genomic predictive abilities (5–8% higher than individual TP prediction), while the effect of including significant QTLs in prediction models was relatively minor (<1–4% improvement). interval mapping) had superior detection power for transient QTLs (i.e. In contrast, even very simple individual TP approaches (e.g.
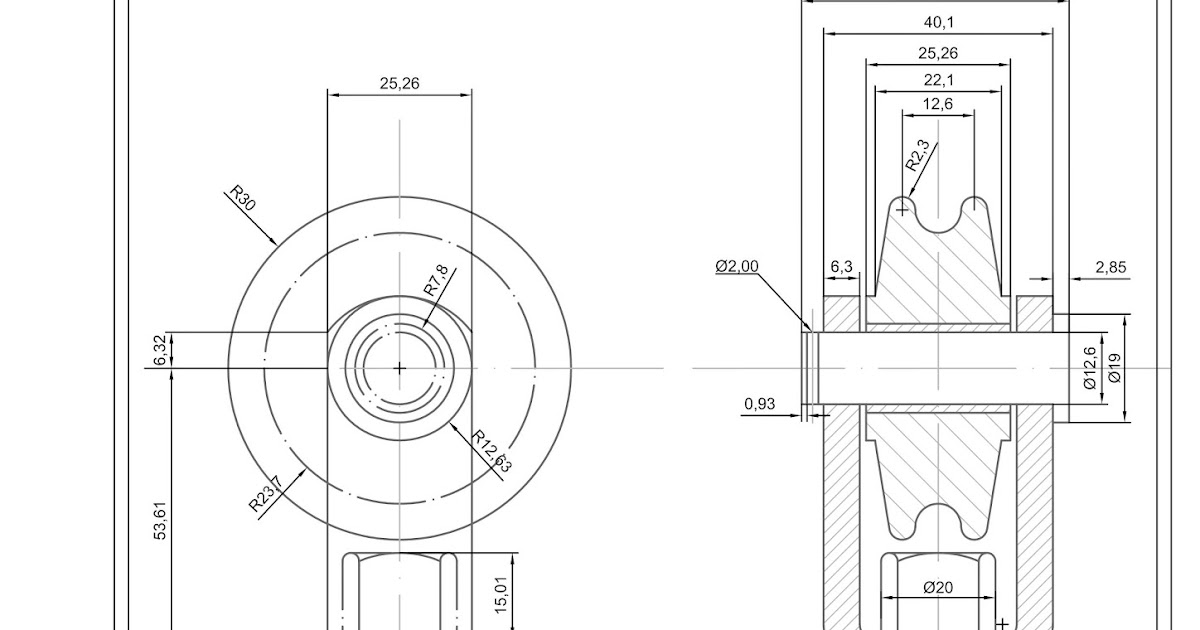
expressed for most of the growing season), with both empirical and simulation analyses demonstrating superior statistical power of detecting such QTLs through functional mapping approaches compared with conventional individual TP analyses. We used canopy height data from an automated field phenotyping platform to compare several approaches to scanning for quantitative trait loci (QTLs) and performing genomic prediction in a wheat recombinant inbred line mapping population based on up to 26 sampled time points (TPs). Genetic studies increasingly rely on high-throughput phenotyping, but the resulting longitudinal data pose analytical challenges.
